Unlocking the Power of Image Annotation in Machine Learning
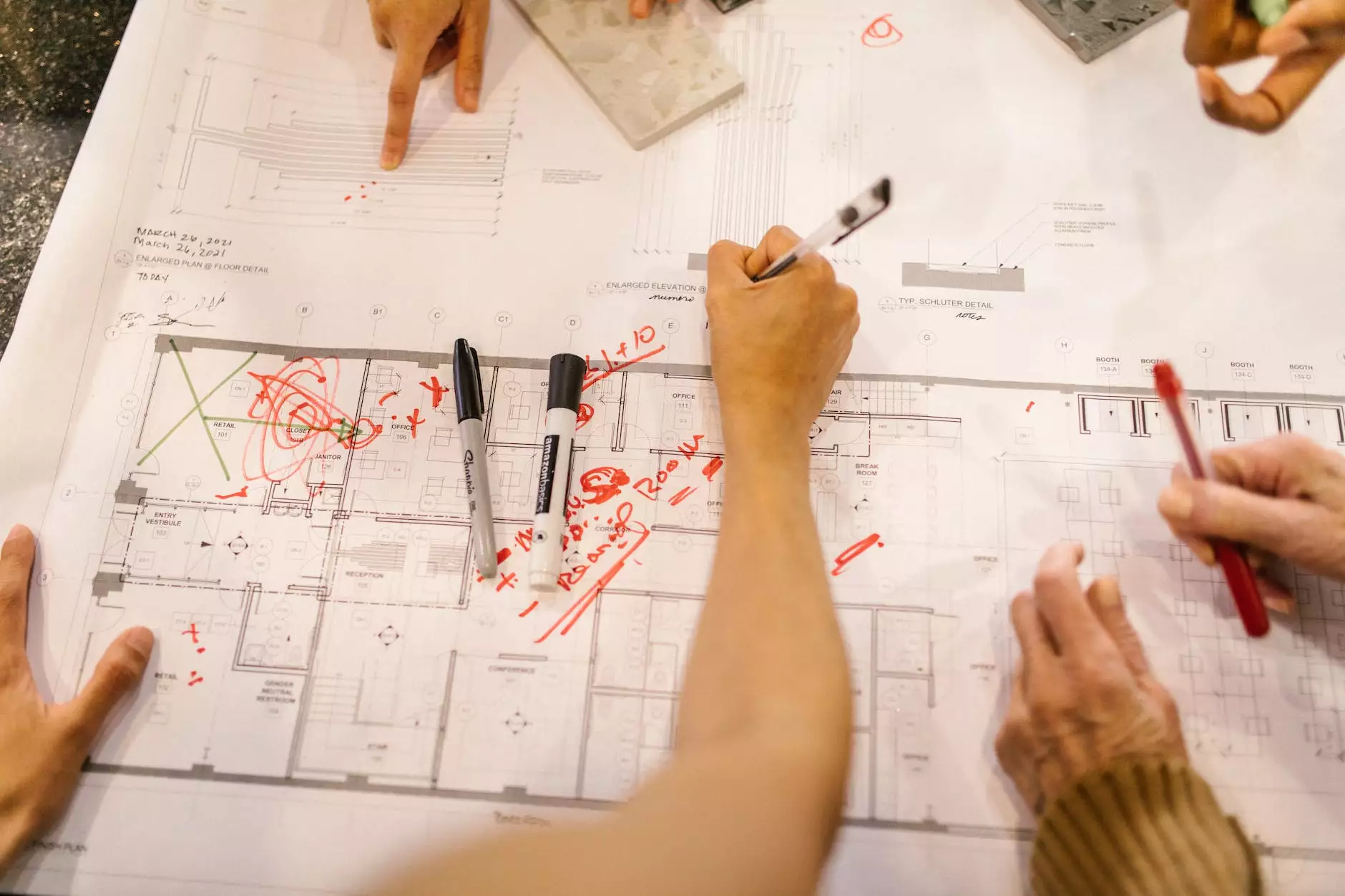
Image annotation machine learning plays a critical role in a variety of industries, particularly as the demand for high-quality visual data continues to grow. This guide explores the profound impact of image annotation technology, the tools and platforms available, and how companies like Keylabs.ai are leading the charge in this vital field.
Understanding Image Annotation
Image annotation is the process of labeling images to provide meaningful information for machine learning models. By adding contextual data to images, algorithms can better understand and interpret visual content, ultimately leading to superior decision-making capabilities.
The Importance of Image Annotation in Machine Learning
In the realm of machine learning, particularly in computer vision, annotated images serve as a training foundation for algorithms. These annotations help machine learning models to:
- Detect Objects: Identify and delineate various objects within an image.
- Recognize Patterns: Understand complex visual patterns that change over time.
- Enhance Accuracy: Reduce errors during the model's prediction phase.
Categories of Image Annotation in Machine Learning
There are several categories within image annotation, each serving unique purposes and methodologies:
- Bounding Box Annotation: This involves drawing rectangles around the relevant objects to identify their location and size within the image.
- Semantic Segmentation: In this method, the image is divided into segments, allowing for more detailed analysis and understanding of the visual content.
- Instance Segmentation: This advanced technique not only segments the image but also differentiates between separate instances of the same class of objects.
- Landmark Annotation: This is used primarily for face recognition, where specific points on a face are annotated to improve identification accuracy.
Keylabs.ai: A Leader in Data Annotation Tools
At Keylabs.ai, we recognize the significance of high-quality data annotation tools in the process of image annotation for machine learning. Our platforms are designed to cater to diverse industry needs by providing:
- Efficient Tools: Our tools streamline the annotation process, making it faster and more accurate.
- Scalability: Whether you have a few images or millions, our platform scales to meet your needs.
- Custom Solutions: We offer tailored annotation solutions that meet the specific needs of your projects.
Advantages of Using Keylabs.ai for Image Annotation
Utilizing Keylabs.ai for your image annotation machine learning needs provides numerous advantages:
- Expertise: Our team consists of experienced annotators who understand the nuances of various industries.
- Quality Control: We implement rigorous quality control processes to ensure the highest level of accuracy in annotations.
- Cost-Effective Solutions: We offer competitive pricing while maintaining high-quality standards.
Image Annotation Process
The image annotation process at Keylabs.ai can be broken down into several simple steps:
- Requirement Analysis: Understanding the specific requirements of the project.
- Image Collection: Gathering the images that need to be annotated.
- Annotation: Trained professionals begin the labeling process using our intuitive annotation tools.
- Quality Assurance: Each annotated image goes through a quality check to ensure it meets standards.
- Delivery: The final annotated data is delivered in the required format for machine learning integration.
Real-World Applications of Image Annotation Machine Learning
The applications of image annotation are vast and varied across multiple industries:
Healthcare
In the healthcare sector, image annotation for machine learning is utilized to enhance diagnostic accuracy. For instance, annotated medical images are used to train models that assist radiologists in identifying anomalies.
Automotive
Autonomous vehicles rely heavily on image annotation. Datasets annotated with bounding boxes around pedestrians, traffic lights, and road signs help to train machine learning models to navigate safely.
Retail and E-commerce
In the retail sector, image annotation aids in visual search capabilities, allowing customers to search for products using images, enhancing the overall shopping experience.
Agriculture
Farmers use image annotation to classify crops and identify disease or pest infestations through machine learning techniques, thereby improving yield and sustainability.
Challenges in Image Annotation
While the benefits of image annotation in machine learning are numerous, several challenges still exist:
- Data Privacy: Safeguarding sensitive data during the annotation process is crucial.
- High Costs: The resource-intensive nature of high-quality annotations can lead to increased project costs.
- Quality Consistency: Maintaining uniformity in quality across large datasets is challenging but essential.
Future of Image Annotation in Machine Learning
The future of image annotation machine learning looks promising, with several notable trends emerging:
- Integration of AI: Utilizing AI to assist human annotators is expected to improve efficiency and reduce costs.
- Active Learning: This approach allows models to identify which examples they struggle with, focusing human effort where it's most needed.
- Cloud-Based Platforms: As cloud technology evolves, it will further democratize access to powerful annotation tools.
Conclusion
In conclusion, image annotation machine learning is not just a technical necessity but a transformative force across various sectors. Companies like Keylabs.ai are pioneering the way forward, offering innovative data annotation tools and platforms that meet the demands of a rapidly evolving market. As industries continue to leverage the power of machine learning, the importance of high-quality image annotation will only grow, reinforcing the need for dedicated and proficient services.
By investing in advanced annotation technologies and platforms, businesses can unlock the full potential of their data, ensuring accurate, efficient, and impactful machine learning applications. The journey of image annotation is just beginning, and the future is vibrant with possibilities.