Harnessing the Power of Machine Learning for Spam Detection
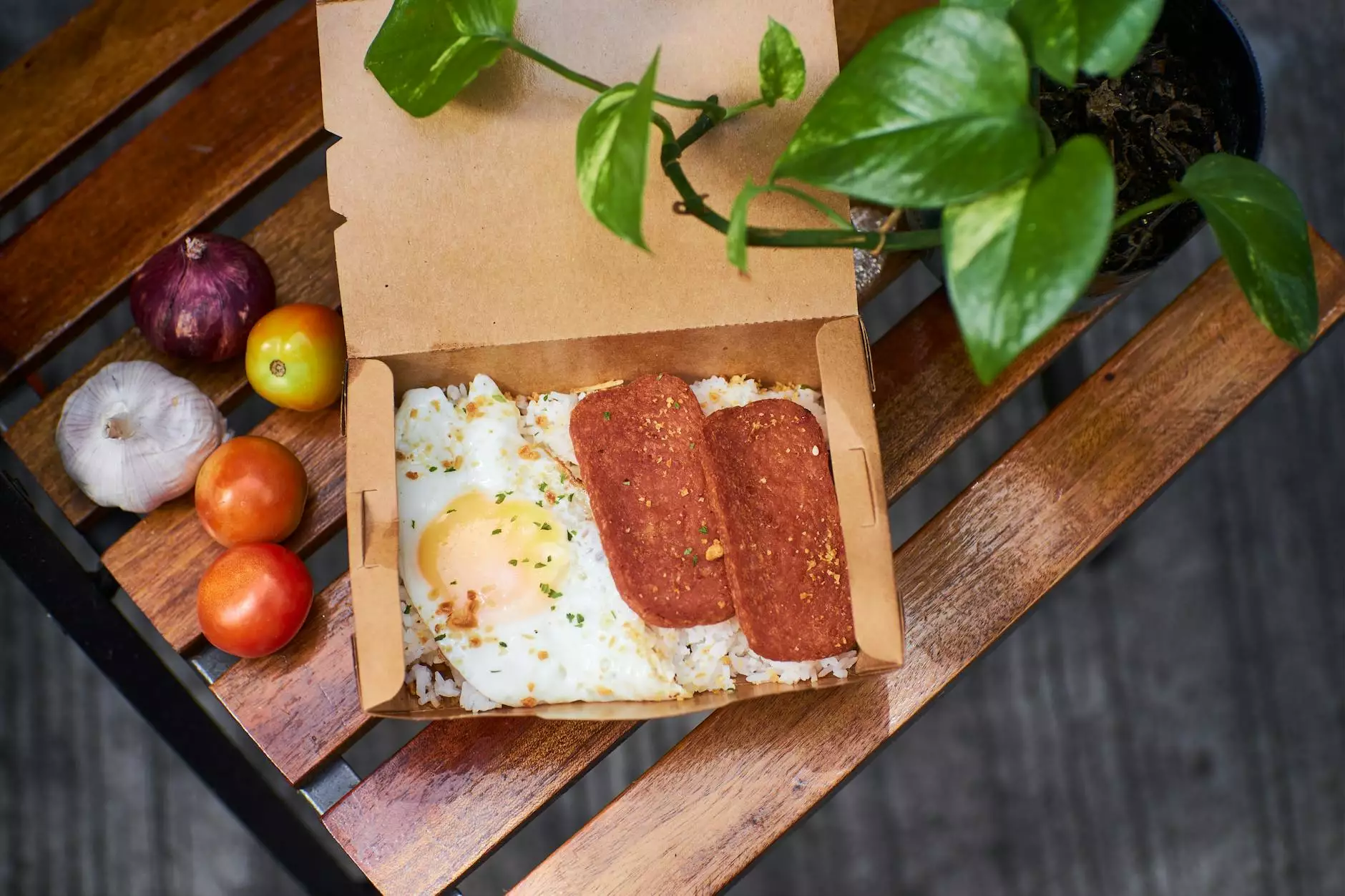
Understanding Spam Detection
In today's digital world, spam has evolved into a major issue for both consumers and businesses. Spam messages can overwhelm inboxes, disrupt workflows, and pose serious security threats. To combat this, innovative technologies like machine learning spam detection have emerged, redefining how businesses manage and protect their digital communications.
What Is Machine Learning?
Machine learning is a subset of artificial intelligence (AI) that allows systems to learn from data, identifying patterns and making decisions with minimal human intervention. Unlike traditional programming, which relies on explicit instructions, machine learning algorithms improve their performance over time by analyzing large datasets. This adaptability is particularly effective in spam detection.
The Importance of Machine Learning in Spam Detection
Traditional spam filters primarily rely on rule-based systems, which are limited in their capabilities. These filters can become outdated quickly, unable to adapt to new spam tactics. Here's where machine learning becomes invaluable:
- Adaptability: Machine learning algorithms continuously learn from incoming data, improving accuracy and reducing false positives.
- Pattern Recognition: Algorithms can identify complex patterns that may indicate spam, using feature extraction techniques to analyze the content, sender, and even the time of sending.
- User Personalization: By learning individual user behavior, machine learning models can tailor spam detection criteria to better suit each user’s preferences.
How Machine Learning Spam Detection Works
The process of implementing machine learning spam detection involves several stages:
- Data Collection: Collecting a large dataset of emails, which includes both spam and legitimate messages.
- Data Preprocessing: Cleaning the data to remove any irrelevant information and standardizing formats.
- Feature Extraction: Identifying key features that help in distinguishing between spam and non-spam emails, such as keywords, phrases, sender reputation, and engagement metrics.
- Model Training: Using the preprocessed data to train machine learning models. Common algorithms include Naive Bayes, Support Vector Machines, and neural networks.
- Model Evaluation: Testing the model’s performance using metrics like precision, recall, and F1 score to ensure it effectively minimizes false positives.
- Deployment: Integrating the model into the email system to filter incoming messages in real-time.
Benefits of Machine Learning in Businesses
Integrating machine learning spam detection into business practices yields numerous benefits, including:
- Enhanced Security: With better filtering capabilities, businesses can protect themselves from potential phishing attacks and malware.
- Increased Efficiency: Employees spend less time sorting through spam, allowing them to focus on productive tasks.
- Cost Savings: Automated spam detection reduces the need for extensive IT support to manage spam-related issues.
- Improved Customer Experience: Clients and customers receive better email communication without the clutter of spam.
Implementing Machine Learning Spam Detection in IT Services
For companies like Spambrella, which operates in the IT Services & Computer Repair category, implementing machine learning for spam detection is vital. Here are actionable steps:
- Assess Current Systems: Evaluate existing spam detection systems to identify gaps.
- Invest in Data Infrastructure: Ensure robust data storage and processing capabilities to handle large datasets.
- Train Staff: Equip the IT team with knowledge about machine learning technologies and their application in spam detection.
- Partner with Experts: Collaborate with machine learning specialists to design and deploy effective spam detection models.
- Monitor and Optimize: Continuously monitor the effectiveness of the spam detection system, making adjustments as necessary based on user feedback and emerging spam trends.
The Future of Spam Detection in Security Systems
As cyber threats evolve, the role of machine learning in spam detection will become increasingly critical. Future innovations may include:
- Deep Learning: Utilizing neural networks to achieve even higher levels of accuracy in recognizing complex spam patterns.
- Real-time Threat Intelligence: Leveraging collective data across various platforms to provide immediate updates on emerging spam tactics.
- Integration with Other Security Systems: Creating comprehensive security systems that combine spam detection with broader network security measures.
Conclusion
In summary, machine learning spam detection represents a significant advancement in the battle against spam. The integration of these technologies not only enhances security but also contributes to greater operational efficiency and customer satisfaction. For businesses operating in the IT services and security systems sectors, embracing machine learning is not just an option—it is a necessity to stay ahead in a rapidly changing digital landscape. By investing in robust machine learning strategies, companies like Spambrella can lead the charge in offering superior spam detection solutions, safeguarding their operations, and delivering outstanding service to their clients.
© 2023 Spambrella - All Rights Reserved.